Written by Kaitki Agarwal, Founder, President and CTO at A5G Networks, Inc. for Forbes.com
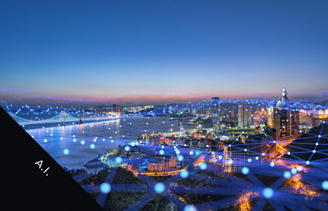
We live in a connected world and use many intelligent devices today, but our world is not as connected and intelligent as we want it to be to enrich our daily lives.
The proliferation of the IoT, smart devices, edge computing and the adoption of virtual reality (VR) and augmented reality (AR), however, are all likely to shape how we live and work in the coming years. As networks evolve and improve, they could bring many benefits and opportunities to consumers and businesses. For example, these evolutions could bring these benefits.
VR/AR: 5G technology is well-suited to support VR and AR technologies and meet the low-latency requirements of VR and AR. This could enable VR and AR for various applications. VR and AR could allow students to take virtual field trips or interact with simulations and simulations of historical events, making learning more interactive and engaging. VR and AR could also provide realistic simulations for workers to practice and develop expertise in a safe, controlled environment.
Industry 4.0: Industry 4.0 involves the development of “smart factories” that can optimise production processes and improve efficiency, quality and flexibility. By increasing the use of IoT devices and sensors, manufacturers could gain greater visibility and control over production processes, which can enable predictive maintenance and the optimisation of production scheduling and resource allocation.
Edge Computing: Edge computing means processing data at or near the point of origin/consumption rather than in a central location. This process can help reduce latency and improve speed, enabling data privacy, security and processing efficiency. Edge computing also enables distributed deployment architectures for 5G. Data collection and data analysis can also be done at the edge for faster results.
The Role Of Autonomous Networks
Edge-based deployment architectures are essential for industry 4.0/private networks, smart cities, connected car networks and public mobile networks. Many of these deployments will involve a variety of deployment architectures, such as on-premise, hybrid and SaaS-based using multi-cloud (private and public).
As the network becomes complex and distributed, though, enabling autonomous and secure connectivity layers to realise these networks becomes increasingly important.
There are many cost drivers for mobile networks and new networks, such as private networks, smart cities and connected car network deployments. Several factors need to be considered to drive the cost down in these networks. With the changed landscape—where mobile deployments involve cloud-native infrastructure—the key is to minimise capex and keep opex low.
AI/ML-enabled network functions will likely play a key role there. AI/ML may be used for automation of network operations (e.g., provisioning, optimisation, fault prediction), security (DDoS and fraud detection), intelligent network slicing, improving the quality of service and customer experience. This will be essential for reducing opex in a distributed, multi-cloud network.
The Realization Of Autonomous Networks
The network function can only follow an AI-native approach, however, when each network function is designed with AI/ML-based decision making as an implicit design principle and not as an afterthought.
3GPP, for example, had developed a logical function called “Network Data Analytics Function” (NWDAF) to provide analytics-related functionality in telecom networks. NWDAF architecture is different from many cloud-based approaches. The AI-native network function approach should align more closely with cloud-based AI/ML infrastructure while achieving the results of NWDAF.
While it is essential to have AI-native network functions, there are some challenges to their adoption. One of the bigger challenges is the compute requirement in the data centre. Basically, data centres should be built with an AI-first approach because that is where these network functions will be running. For a significant total cost of ownership reduction, including energy savings, AI and networking costs need to be brought down significantly. Some of these efforts are already underway by chip vendors as well as server vendors.
Another challenge is the evolving technology for federated AI. For complete adoption of distributed network function with AI, federated ML will play an important role. There are innovations happening in this area, but it may take some time to fully evolve as a commercial solution. The industry may take a phased approach here and create a tightly coupled federated ML to enable the distributed AI-native network functions and bring loosely coupled and more generic federated ML in the later phases.
Conclusion
For distributed and multi-cloud-based network function layers, AI/ML could optimise edge data centre resource utilization, elastic scaling, anomaly detection and predictive analytics. In all of these scenarios, data integrity, data governance, data collection and storage and data pipeline management should be considered for distributed networks.
Autonomous networks could also evolve current walled garden mobile operator networks into more open and futuristic networks. Mobile network infrastructure transformation has been challenging and expensive. Mobile operators want to maximise their previous investments and continue using the existing network infrastructure; this spans devices, transport, RAN, core and OSS/BSS.
In fact, taking the incremental improvement approach within existing network may need to be challenged as it may not bring the desired value. Autonomous network functions could make it easier to introduce innovation to enable new revenue and technologies. In some cases, it might be advisable to create new networks and think along the lines of NaaS (network as a service) or CaaS (core as a service).
Click here to read the original article.